AI-based model development for Immuno-informatics
We develop AI algorithms for analyzing massive immunology, multi-omics as well as clinical data to innovate novel personalised immunotherapies
High-throughout data analysis facility
Our customised AI empowered immuno-informatics workflow
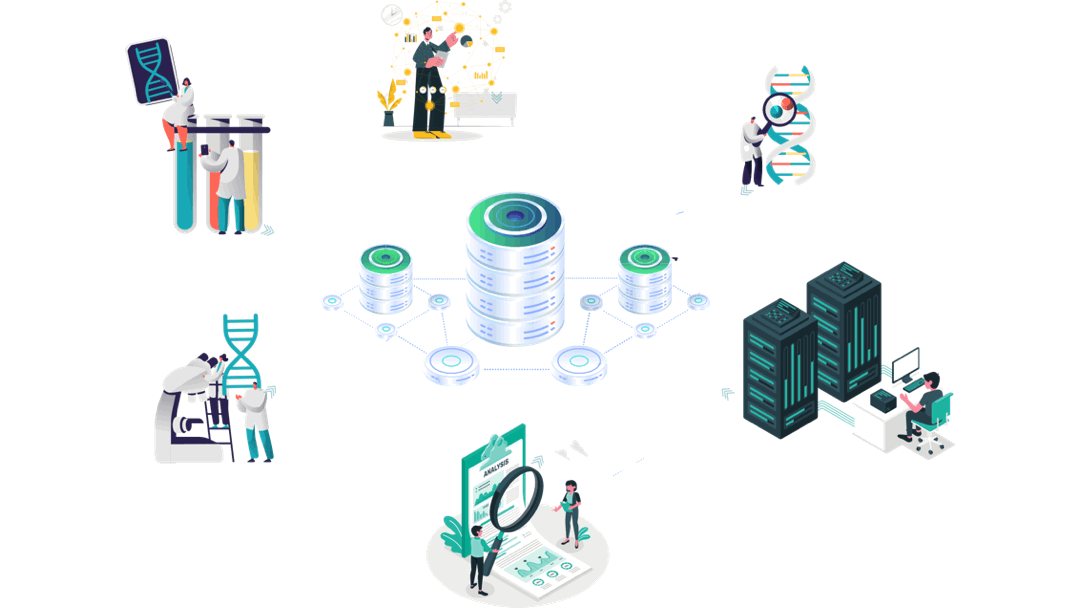
Experimental Data
Immunology/Clinical Data generation
Clinical trials’ data analyses
Aomics core facility:
- Artificial Intelligence model development
- Scientific Data mining/processing
- Analytical workflows development and maintenance
Patient data collection
Omics analyses
Artificial intelligence & Machine Learning for Immuno-informatics
We develop powerful and innovative AI models and pipelines for Immuno-informatics. By leveraging AI, we assist making powerful insights from the high-throughput immunological data.
We use artificial intelligence (AI) and machine learning (ML) techniques to optimize the properties of therapeutic antibodies. Therapeutic antibodies are antibodies that are developed for use in treating diseases, such as cancer and autoimmune disorders.
Optimizing the properties of therapeutic antibodies is important for improving their efficacy and reducing potential side effects. Antibody optimization using AI involves the use of computational methods to predict the effects of changes to the antibody structure on its properties, such as its affinity for its target and its stability.
AI and ML techniques can be used to analyze large datasets of antibody structures and properties to identify patterns and relationships between different variables. These techniques can then be used to develop predictive models for optimizing antibody properties.
Immune modulators are molecules that can stimulate or suppress the immune system, and they have potential applications in the treatment of various diseases, including cancer, autoimmune disorders, and infectious diseases.
One approach for using AI in immune modulator discovery is to employ virtual screening techniques to identify molecules that can interact with specific immune system targets. Virtual screening involves using computational methods to screen large libraries of compounds to identify those that are most likely to bind to a target of interest. Machine learning algorithms can be used to predict the binding affinity of potential modulators based on their molecular structure.
Another approach is to use AI to analyze large datasets of biological and clinical data to identify potential immune modulators. For example, machine learning algorithms can be used to analyze gene expression data from patient samples to identify genes that are differentially expressed in patients with a particular disease. These differentially expressed genes could represent potential targets for immune modulator development.
AI algorithms can be used to analyze large-scale immunological data sets and predict immune responses to specific pathogens or immunotherapies.
AI can assist in the design of novel vaccines by analyzing the interaction between the immune system and pathogens, predicting potential epitopes, and optimizing vaccine formulations.
AI can assist in the identification of potential epitopes for vaccine development by analyzing large-scale immunological data sets and predicting antigenic regions of proteins.
AI can assist in the discovery of new immunomodulatory drugs by analyzing the immune system’s response to pathogens and predicting potential targets for drug development.
AI can assist in the analysis of the immune repertoire by analyzing large-scale sequencing data and identifying unique features of immune cells in response to different stimuli.
Single-Cell Analysis for understanding Spatial Heterogeneity
Single-cell analysis is a valuable tool for understanding the spatial heterogeneity of the tumor microenvironment (TME). The TME is a complex and dynamic ecosystem composed of cancer cells, immune cells, stromal cells, and extracellular matrix components that interact with each other and shape the tumor's growth and progression. Single-cell analysis techniques, such as single-cell RNA sequencing (scRNA-seq) and imaging mass cytometry, can be used to analyze the gene expression and protein levels of individual cells within the TME. These techniques enable the identification of different cell types, such as tumor-infiltrating immune cells, cancer-associated fibroblasts, and endothelial cells, and can reveal the cellular heterogeneity within the TME.
Single cell sequencing for studying the immune system
Single-cell sequencing is a powerful tool for studying the immune system at the single-cell level. The immune system is composed of diverse cell types, including T cells, B cells, natural killer cells, dendritic cells, and macrophages, which work together to defend the body against pathogens and other threats. Single-cell sequencing techniques, such as single-cell RNA sequencing (scRNA-seq), can be used to analyze the gene expression of individual immune cells. This enables the identification of different immune cell types, as well as the identification of subsets and states within each cell type. For example, scRNA-seq can reveal the different types of T cells, such as CD4+ helper T cells and CD8+ cytotoxic T cells, and the different subsets of these cell types, such as memory T cells and regulatory T cells.
Artificial intelligence & Machine Learning for Immuno-informatics
Artificial intelligence (AI) and machine learning (ML) techniques are increasingly being used in immuno-informatics to analyze and interpret large amounts of data generated from high-throughput experiments. Immuno-informatics involves the application of computational and informatics methods to study the immune system, including the analysis of immune responses, vaccine design, and immunotherapy development. AI and ML techniques can be used to analyze high-dimensional datasets generated from techniques such as single-cell sequencing, mass spectrometry, and flow cytometry. These techniques can identify complex patterns and relationships between variables in the data that may be difficult or impossible to detect through manual analysis.
How we use Multi-omics data for Immuno target identification?
Multi-omics data integration is a powerful approach for identifying potential targets for immunotherapy development. Multi-omics data refers to the integration of multiple types of molecular data, such as genomics, transcriptomics, proteomics, and metabolomics, to provide a more comprehensive view of the molecular mechanisms underlying a disease or biological process. In the context of immuno target identification, multi-omics data integration can be used to identify potential targets for immunotherapy development. For example, by integrating genomics and transcriptomics data from patient samples, researchers can identify genes that are differentially expressed in tumor cells compared to normal cells. These differentially expressed genes may represent potential targets for immunotherapy development.
Would you like to start a project with us?
Reach out on the given number or write us an email for further queries regarding project requirements